How The Forecasting Methods Are Evolving For Supply Chain Optimization?
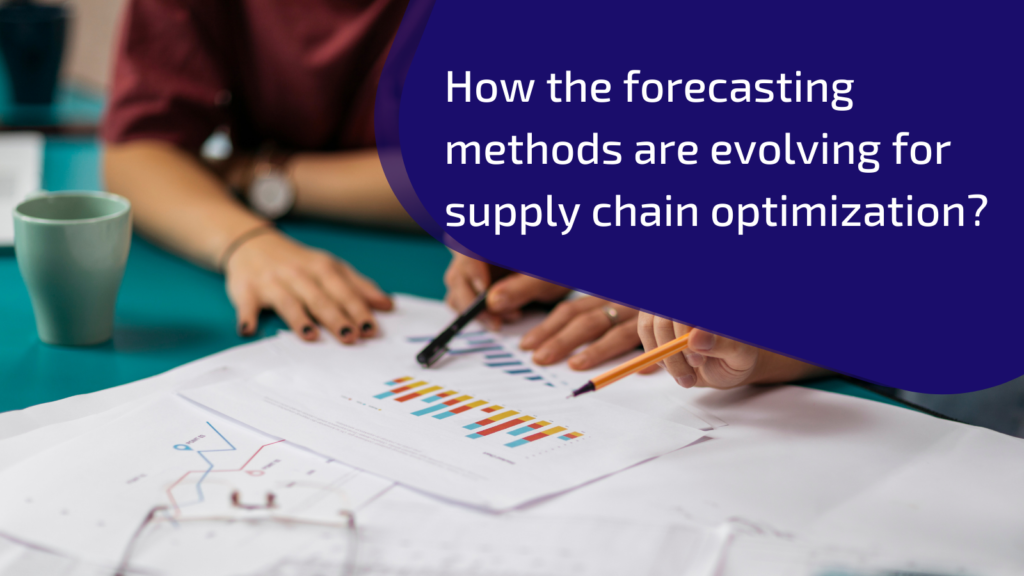
Supply chain forecasting combines data from past supply with insights and understandings about demand, to help you make the best decisions for your organisation– whether it’s stock inventory, cargo booking, budget planning or expanding to new markets.
It involves looking at data & information about your suppliers to understand when you need to order products from them – whether they’re whole products or raw materials to be assembled further down the supply chain.
Methods of forecasting – Quantitative
There are two important methods to producing supply chain forecasting – quantitative and qualitative. Quantitative forecasting depends on historical data to predict future sales, making use of complex algorithms and computer programs to do so.
In quantitative forecasting, you might follow the below methods. Each has its benefits and disadvantages and should be considered carefully to determine their best use:
- Moving average forecasting is one of the easier methods of forecasting, which is based on historical averages. However, it treats all data equally and doesn’t take into account that more recent data may be a better indicator of coming trends than say, data from three or five years ago – and it doesn’t allow for seasonality or trends.
- Exponential smoothing also considers historical data but does put more focus on recent data – as well as accounting for seasonality. This makes it perfect for short-term forecasts.
- Auto-regressive integrated moving average (ARIMA) is a method of forecasting that is known for being highly accurate and precise, but also very time-consuming and costly. It is suitable for forecasting up to 18 months or less.
- Multiple Aggregation Prediction Algorithm (MAPA) is a newer method of quantitative forecasting which is specifically designed for seasonality – which makes it perfect for businesses producing seasonal items.
Methods of forecasting – Qualitative
In the event of historical data being hard to find, when launching a new product, for example, you need a new approach – and that’s where qualitative forecasting comes in useful. It depends on the insights, expertise and experience of industry experts – alongside more detailed research:
- Historical analogies predict sales by assuming that the sales of new products will mirror a current product that you, or a competitor, produces. While it can operate in the long term, it’s not advised for short-term forecasting.
- Internal insights are a ground-up approach to forecasting; using the insights and opinions of experienced staff members to inform predictions. As you might expect, it isn’t known for a high level of accuracy but is an option when quantitative methods aren’t possible.
- Market research will be familiar to many organisations and is the process – sometimes timely and costly – of researching, surveying, polling or interviewing a specific demographic.
What is the best method of supply chain forecasting?
There is no one-size-fits-all approach to supply chain forecasting – and regardless of which you choose, you will never be 100% accurate and precise – because at least some of the forecasting is based on assumptions; and there will always be unforeseen events that defy those assumptions – like a pandemic, for instance!
However, while qualitative forecasting has its place in those situations where historic data is unavailable, it is the consensus that quantitative forecasting is the strongest technique. It uses significant information and statistical techniques, which removes the risk of bias while producing clearer and more accurate results.
What makes supply chain forecasting difficult?
Supply chain forecasting extends a world of opportunities for your business through the access it provides to insights on future demands, trends and supply information. But there are several factors that can disrupt the system.
- Regulation changes and global events like COVID-19 – Changes in regulations between nations and continents can often disrupt forecasting as supply chains adjust to accommodate new laws and past data becomes a little less relevant. Due to COVID-19, emergency laws were passed around the world to close borders, stop travel and delay trade – the impact has been far-reaching and ongoing, with bottlenecks at borders and congestion at ports. Combined with Brexit, it’s easy to see how regulation changes can disrupt & break supply chains and supply chain forecasting.
- Changing trends and consumer habits – While changing trends and habits are a constant in the supply chain management sector, the unpredictability with which they change means they remain a threat to forecasting. For instance, in the last year as the world closed down consumers went online and spent over $4.2 trillion dollars worldwide – while online shopping has only been growing, the sudden shift forced many small businesses to adapt quickly to avoid stock shortages or delays.
- Seasonality and supplier lead times – Not accounting for seasonal and peak periods in supply chain forecasting will easily toss your forecasting off the course. These periods in the calendar often impact ocean freight and should be planned months in advance – or you risk missing out on opportunities to capitalize on increased demand.
An important aspect to consider when doing this planning is that different suppliers or manufacturer will have different lead times, not just based on the services they provide, but due to their own seasonal or holiday calendars. This drives building strong relationships with suppliers really important.
The rise of AI and ML technologies provides demand planners with incredibly powerful tools and insights, but demand planners will need to develop skills in supply chain understanding models. They should understand how to extrapolate the ML model rather than how to build ML models. This skill set is required to make predictions & forecasts, figure out why they are correct and effectively control supply and demand. Companies that take an AI-first approach can rapidly develop and deploy agile and accurate supply chain solutions for demand forecasting, built with technologies that can automate redundant tasks and the entire data journey. A 5% increase in forecast accuracy can improve the profitability up to 1%, adding millions of dollars to the bottom line by focusing on data-driven planning.
The two main trends in forecasting that have largely benefited organizations to recover and thrive in the post-pandemic world are automation and scenario planning.
Automation
AI solutions can help eliminate large amounts of work and help connect large amounts of data produced within an organization quickly and efficiently. Automation can allow businesses to integrate their analytical models into the production environment for predictive analysis.
Bring in low-touch/no-touch demand planning powered by AI, which can help in setting up accurate demand sensing and shaping processes in all under 3 weeks and also helps you stay prepared with features such as scenario modelling and real-time collaboration, which can help in preparing for various scenarios in these testing times to keep your business optimised while helping you come up with plans accurate plans, fast.
As a result, this assists businesses to change from a traditional to a sophisticated demand planning culture.
Scenario planning
It is critical to plan for multiple scenarios in order to reflect upon any uncertain situation that may come up. Supply chain executives can see how demand fluctuates for each SKU at each location based on historical data and various socioeconomic factors by simulating any possible what-if scenarios. The supply chains that remained stable during the lockdown depended heavily on scenario planning. This will be a key trend as we move into an uncertain future with volatile supply and demand.
Implementing agile forecasting solutions
2020 saw an acceleration of digitization of various supply chain processes, but most companies fail to effectively implement AI, and demand planning teams end up retreating to more familiar and traditional forecasting methodologies.
The implementation process is filled with lengthy, inconvenient decision-making processes, redundancies, complex workflow, and poor KPI evaluation and missed opportunities as a result of the lengthy implementation process.